Introduction
The previous section provided an overview of the updated Life Cycle Analysis (LCA) conducted for the school’s components. This updated LCA considers the material quantities needed for a school building based on its specific length, width, and height. Additionally, it takes into account the additional material quantities required for specific maintenance interventions. In the scope of this LCA, each material is evaluated based on the associated primary energy demand, global warming potential and cost.
In this section, we introduce a Multi Objective Optimization process. Unlike the section on Maintenance Strategies, which focused on overall downtime and frequency of maintenance interventions as performance criteria, this section aims to provide a more comprehensive approach to evaluating the system. This is crucial because each maintenance activity not only impacts the system’s downtime but also affects the primary energy demand, global warming potential, and cost associated with material production and labor expenses. In order to assess maintenance strategies comprehensively, these performance criteria must be taken into consideration.
Design Parameters and Performance Criteria
Selection of Design Parameters and Performance Criteria are essential for obtaining a holistic view of the system under examination. The optimization process will incorporate, as design parameters, the required maintenance events and their frequencies [years]. Additionally, system design parameters such as height[m], width[m], and length[m] of the school will be considered to assess their relationship with an optimal strategy. Figure 1 provides an overview of the value range, for each design parameter.
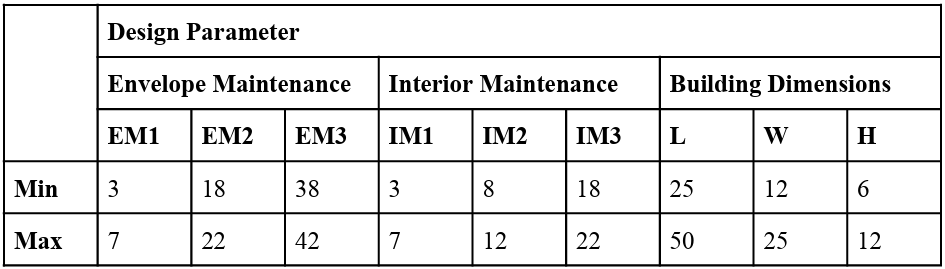
The performance criteria to be evaluated encompass the overall system downtime and minimum frequency of interventions. On the other hand, the primary energy demand, global warming potential, and cost associated with the resulting material consumption will be evaluated as well.
Optimization
Figure 2-3 present results from the optimization process, depicting the pareto fronts for two performance criteria each.
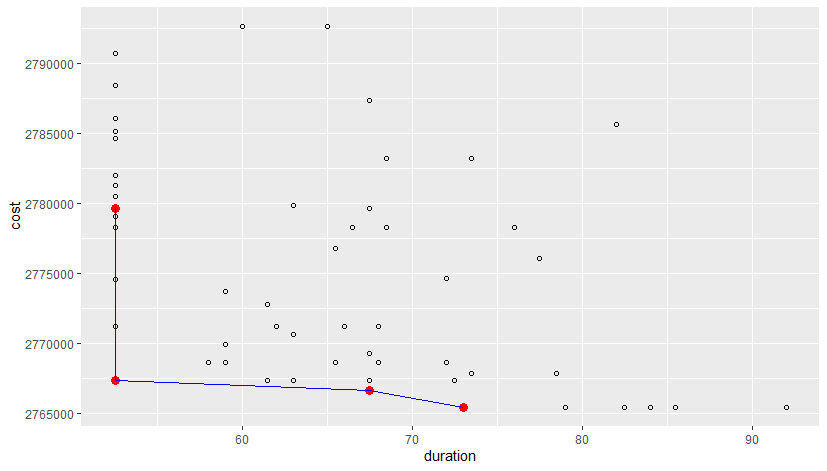
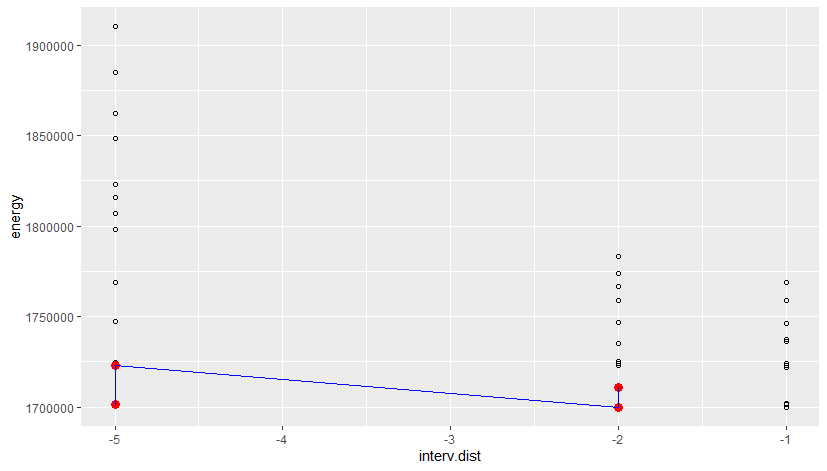
Maintenance Frequency and Duration
When comparing these results with the outcomes of the previous maintenance strategy optimization, we observe that the pareto fronts now include values for system downtime that are significantly larger and intervention frequencies that are significantly lower. This is because the previous optimizations only focused on minimizing downtime and maximizing the time between interventions. However, now that criteria related to material quantities (such as cost, global warming potential, and primary energy demand) are considered, the pareto optima are influenced by these additional performance criteria. This is underlined by the slightly irregular distribution of pareto optima in the plots, which can be explained by the fact that the optimization process considers five performance criteria, whereas each plot only accounts for two of them.
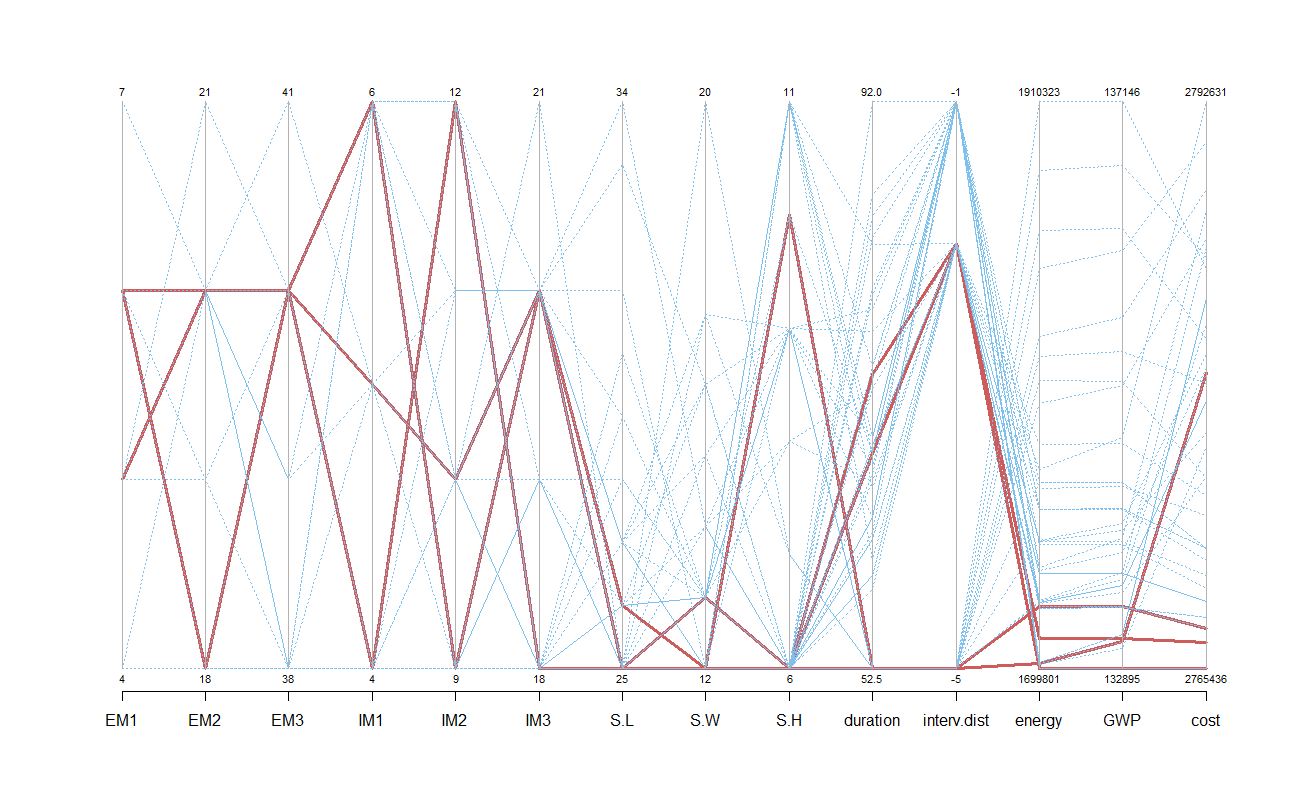
Building Dimensions
Analyzing the pareto optima in relation to the design parameters (Figure 4), we find that for the building dimensions, the optima tend to prefer school length and width closer to the lower bound of the input range. This preference can be explained by the fact that, for schools with the same maintenance frequencies, smaller dimensions result in more efficient material consumption per maintenance event. This, in turn, leads to improved efficiency in terms of primary energy demand, global warming potential, and cost.
Considerations for Maintenance Strategy Planning
Regarding the frequency of individual envelope and interior maintenance events, the pareto optima do not indicate a clear preference. However, it is important to consider various factors when determining the maintenance strategy. In general, overlapping maintenance interventions can reduce downtime if activities can be carried out concurrently. However, it is crucial to avoid carrying out interventions more frequently than necessary, as this can increase life cycle cost, global warming potential, and primary energy demand due to the associated material consumption over the system’s lifetime.